Table Of Content
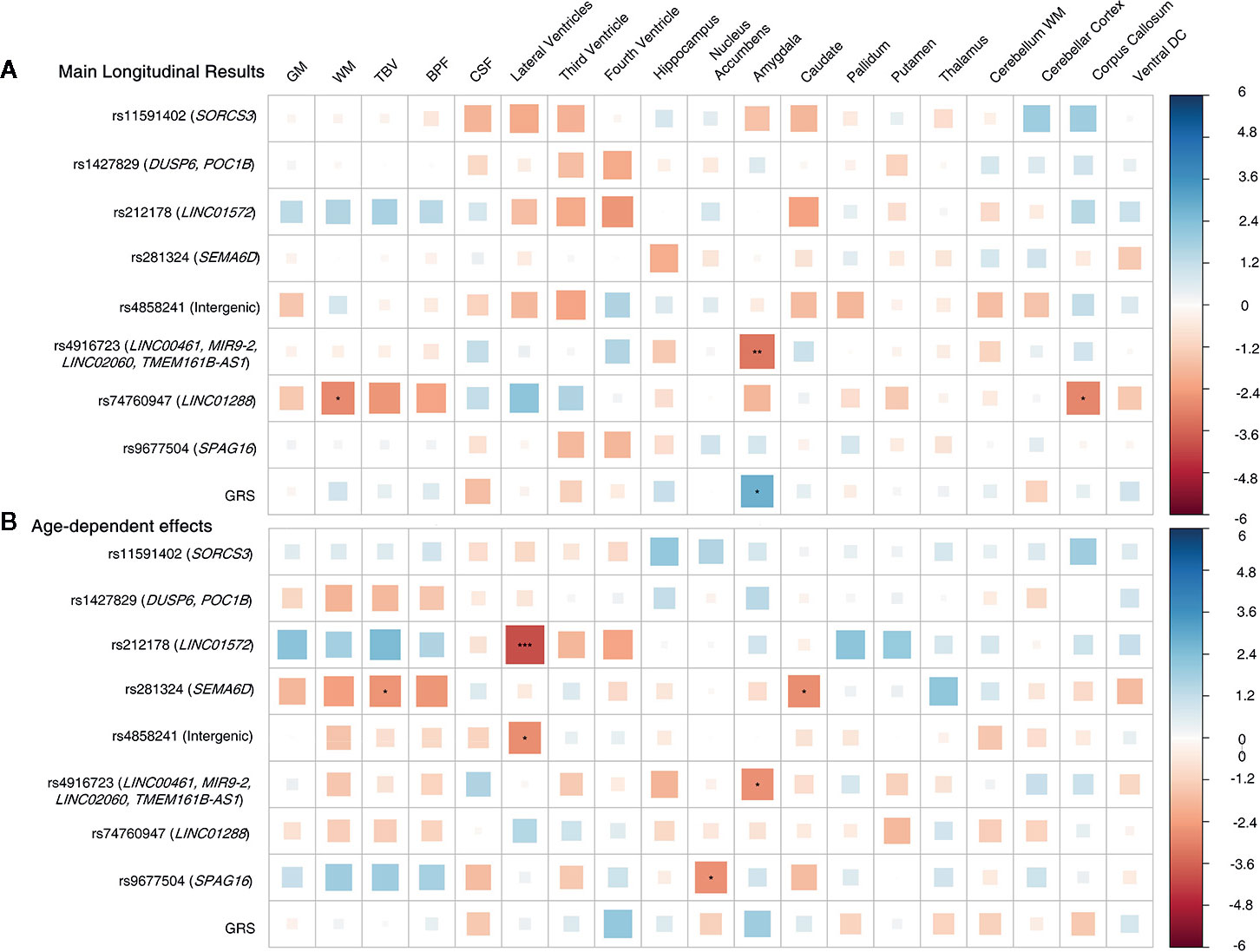
Fortunately, this problem is quickly mitigated by more waves of observations and more cases (i.e., the bias is largely removed with 60 pairs of observations). These statistics are generally very trustworthy and allow you to investigate changes over a long period of time. To preserve the anonymity of the participants, the data collected is often aggregated so that it can only be analysed on a regional level. You will also be restricted to whichever variables the original researchers decided to investigate.
Differences in Stability
Longitudinal changes in high sensitivity C-reactive protein associated with serum uric acid in the Korean Genome and ... - Nature.com
Longitudinal changes in high sensitivity C-reactive protein associated with serum uric acid in the Korean Genome and ....
Posted: Wed, 03 Jan 2024 08:00:00 GMT [source]
If your organization has done these surveys for the past 10 years, you now have more than enough material to conduct a retrospective study. When understanding what is a longitudinal study, it’ll be well worth your while to look into retrospective studies. For your company, retrospective longitudinal studies can reveal crucial insights without you having to spend a single dime. Since these studies depend on existing data, they not only don’t cost much themselves but also improve the returns from your earlier research efforts. The one key difference between cohort studies and panel studies is that the same set of people has to be observed in the latter. In cohort studies, you can pick a different sample of the same demographic to study over time.
Frequently Asked Questions
For example, intervals can be too short, such that given the rate of an effect, the cause might not have had sufficient time to register on the effect. Alternatively, if intervals are too long, an effect might have triggered a compensating process that overshoots the original level, inverting the sign of the cause’s effect. Figure 1 depicts this process, which refers to the response to an emotional stimulus. Specifically, the emotional response elicits an opponent process that, at its peak, returns the emotion back toward the baseline and beyond. If the emotional response is collected when peak opponent response occurs, it will look like the stimulus is having the opposite effect than it actually is having. The statistical testing of longitudinal data necessitates the consideration of numerous factors.
Advantages and disadvantages of longitudinal studies
In these types of studies, researchers tracked participants from childhood into adulthood to see how environment influences personality, achievement, and other areas. So, careful methodology is key throughout the design and analysis process when working with repeated-measures data. In addition to those considerations, optimizing the time lags between measurements, maximizing participant retention, and thoughtfully selecting analysis models that align with the research questions and hypotheses are also vital in ensuring robust longitudinal research. Once you determine the type of longitudinal study you will conduct, you then must determine how, when, where, and on whom the data will be collected. They are beneficial for recognizing any changes, developments, or patterns in the characteristics of a target population. Longitudinal studies are often used in clinical and developmental psychology to study shifts in behaviors, thoughts, emotions, and trends throughout a lifetime.
For example, assume again that individual performance metrics are monitored across time for individuals in high-demand occupations with the first one collected on the date of hire. Assume as well that these individuals are required to report when an external recruiter approaches them; that is, they are not prohibited from speaking with a recruiter but need to just report when it occurred. Due to some cognitive dissonance process, individuals may start to discount the current employer and reduce their inputs.
Genetic epidemiology of resistance to M. tuberculosis Infection: importance of study design and recent findings Genes ... - Nature.com
Genetic epidemiology of resistance to M. tuberculosis Infection: importance of study design and recent findings Genes ....
Posted: Sat, 22 Apr 2023 07:00:00 GMT [source]
The time point is not the state or value of the substantive variable that we are interested in for tracking changes over time. Changes over time occur when the state of a substantive variable changes over different points of measurement. Finally, in a longitudinal study of changes over time, “time” is distinct from the substantive process that underlies the change over time.
Longitudinal studies vs. Cross-sectional studies
Thus, testing and controlling for retest effects are important considerations in longitudinal research. This tendency is known as selective attrition and can threaten the validity of an experiment. For this reason, researchers using this approach typically recruit many participants, expecting a substantial number to drop out before the end. Knowing what information a study should gather is the first step in determining how to conduct the rest of the study. All rights are reserved, including those for text and data mining, AI training, and similar technologies.
Advantages of Longitudinal Studies
In the non-longitudinal variety, participants are repeatedly exposed to different treatments or tasks, but time is considered an irrelevant feature. In fact, the researcher counterbalances the different treatment sequences across participants in an effort to eliminate temporal... Longitudinal methods may provide a more comprehensive approach to research, that allows an understanding of the degree and direction of change over time. One should carefully consider the cost and time implications of embarking on such a project, whilst ensuring complete and proven clarity in design and process, particularly in view of the protracted nature of such an endeavour; and noting the peculiarities for consideration at the interpretation stage. Conducting longitudinal research is demanding in that it requires an appropriate infrastructure that is sufficiently robust to withstand the test of time, for the actual duration of the study.
How to Perform a Longitudinal Study
In this case, researchers would want to observe these participants from childhood to adulthood, to understand how growing up in different environments influences traits, habits, and personality. To address this question, what follows is largely an application of some of the ideas presented by Mitchell and James (2001) and by Ployhart and Vandenberg (2010) in their respective publications. Thus, credit for the following should be given to those authors, and consultation of their articles as to specifics is highly encouraged. Longitudinal studies are time-consuming and often more expensive than other types of studies, so they require significant commitment and resources to be effective. The Critical Appraisal Skills Programme (CASP) (4) offers a series of tools and checklists that are designed to facilitate the evaluation of scientific quality of given literature. Additional depth of quality assessment is available through the use of various tools developed alongside the Consolidated Standards of Reporting Trials (CONSORT) guidelines, including a structured 33-point checklist proposed by Tooth et al. in 2004 (5).
They could also integrate continuous variables and discrete variables, as either predictor or outcome variables, in a single analytical model to describe and explain simultaneous quantitative and qualitative changes over time. In a recent study, my coauthor and I applied an example of a mixture latent model to understand the retirement process (Wang & Chan, 2011). Longitudinal modeling tends to involve a lot of construct- or variable-level missing data (i.e., omitting answers from an entire scale, an entire construct, or an entire wave of observation—e.g., attrition). Such conditions create many partial nonrespondents, or participants for whom some variables have been observed and some other variables have not been observed. Thus a great deal of missing data in longitudinal designs tends to be MAR (e.g., because missing data at Time 2 is related to observed data at Time 1).
As noted earlier, cross-sectional data are easier to get, but they estimate the wrong parameter. Longitudinal research designs can, with certain precautions, improve one’s confidence in inferences about causality. When this is the purpose, time does not need to be measured or included as a variable in the analysis, though the interval between measurements should be reported because rate of change and cause are related.